How Darknet Transactions are Traced using Blockchain Analysis.
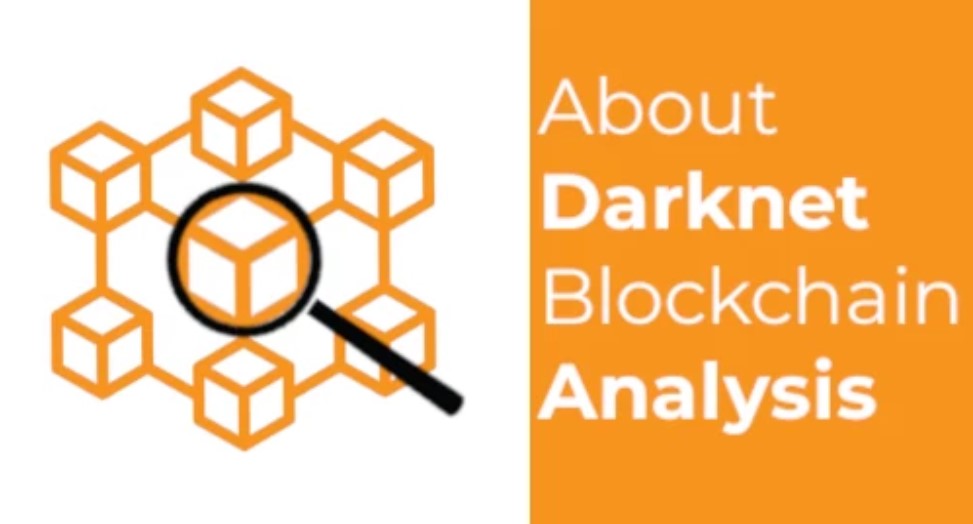
Blockchain analysis is a technique used to track and analyze transactions on a blockchain network. It is particularly used for tracking transactions on the darknet, a network that is hidden from public view and often used for illegal activities. In this article, we will explore the technical details of how blockchain analysis works, how it is used to track darknet transactions, and how to stay protected.
Understanding Blockchain
Before we can dive into the details of blockchain analysis, we need to understand what a blockchain is and how it operates. A blockchain is a decentralized ledger that records transactions in a secure and transparent manner. Each block in the blockchain contains a hash of the previous block, creating a chain of blocks that cannot be altered or deleted. This makes blockchain a secure and tamper-proof method of recording transactions.
The transactions on a blockchain are verified and recorded by a network of computers, known as nodes. These nodes work together to ensure that the blockchain remains secure and that the transactions on it are valid. Once a transaction is added to the blockchain, it cannot be altered or deleted, making it a permanent record.
Time-Series Analysis
Time-series analysis is a technique used to analyze data that changes over time. It is commonly used in blockchain analysis to identify trends in the transaction data over time. For example, time-series analysis can be used to identify when a particular cryptocurrency is being traded more frequently, or when a particular user is more active on the blockchain. Time-series analysis is useful in blockchain analysis because it can help analysts understand how the transaction data is changing over time. By identifying trends in the data, analysts can gain insight into how the blockchain is being used and what factors are driving changes in the transaction data.
For example, let’s say that an investigator is trying to track the transactions of a particular Darknet marketplace over time. By analyzing the transaction data for that marketplace using time-series analysis, they may be able to detect patterns in the volume and frequency of transactions that could indicate changes in the activity of the marketplace.
For instance, a sudden surge in the volume of transactions associated with the marketplace could indicate an increase in demand for illegal goods or services. Similarly, a sudden drop in the volume of transactions could indicate a crackdown by law enforcement or the marketplace shutting down. This technique is mainly used to keep track of dark-net traffic, and identify future targets based on transaction volume.
Clustering Analysis
Clustering analysis is another technique used in data analysis. It involves grouping similar transactions together based on their attributes. For example, clustering analysis can be used to group transactions that have similar values, or that involve the same cryptocurrency address. Clustering analysis is useful in blockchain analysis because it can help analysts identify patterns in the transaction data that are not immediately apparent. This means that it can be used to identify groups of transactions that are associated with a particular user or entity like a specific market.
Statistical Analysis
Statistical analysis is a common technique used in data analysis. it involves using statistical methods to analyze the transaction data on the blockchain. For example, statistical analysis can be used to identify the most active Bitcoin addresses on the blockchain or to determine the average transaction value for a particular cryptocurrency. One popular statistical analysis technique in blockchain analysis is descriptive statistics. It is used to describe and summarize the transaction data. Descriptive statistics can help analysts understand the distribution of transaction values, identify outliers, and detect trends in the data. Some markets now randomize the transaction fee to help overcome this.
Another statistical analysis technique used in blockchain analysis is inferential statistics. This involves using statistical models to infer information about the transaction data from a sample of the data. Inferential statistics can be used to estimate the total value of transactions on the blockchain or to identify patterns in the data that are not immediately apparent. For example, if a user is consistently involved in transactions that are much larger than the average transaction value for that cryptocurrency, it could be a sign that they are engaged in illegal activities such as money laundering or illicit trading.
Machine Learning Analysis
Another technique used in blockchain analysis involves machine learning. This is done by training a computer model to recognize patterns in the transaction data on the blockchain. Machine learning algorithms can be used to identify patterns and trends that are not immediately obvious to human analysts. This is a very important technique and will play a huge role in the future of blockchain analysis. This is the case as machine learning can be used to identify patterns and analyze volumes of data not possible for humans. It is unclear how exactly these models are structured and what data they use as most of this information is highly classified.
Staying protected
In the field, law enforcement combine some of these techniques to track down illicit crime groups and networks. The most common way involves grouping and tracking tracing. Large number of transactions and addresses are grouped together and linked to a criminal network. Individual transactions can then be traced all the way back to their origin. With the rise of bitcoin mixers, and privacy coins most of these techniques are becoming obsolete by the day.
By using privacy coins like monero users can stay securely shop on the dark-net. Their private blockchain nature makes them nearly impossible to track. However there still are a few ways to track privacy coins but it’s not simple. Let’s say you buy some monero on an exchange using your ID, and then deposits it to a dark-net there market. Law enforcement can access exchange data, meaning they can identify where the funds were sent. For this technique to work the market typically need to be compromised. A simple way to overcome this is by sending the coins through a at-least 2 of cold wallets and then to the market, this way the origin is permanently hidden.
Conclusion
In conclusion, blockchain analysis consists of powerful tools that law enforcement agencies and other organizations use to track and monitor transactions. By using a variety of statistical and analytical techniques, such as data analysis and time-series analysis, investigators can identify patterns and trends that may indicate suspicious or criminal activity, and use this information to build cases and hold criminals accountable. As blockchain technology continues to evolve, it becomes harder and harder to track such transactions. Despite that law enforcement continues to evolve and develop new technique to track users.